Devine at Sloan 2014: Automated decision-making: The machines don't know it all, but they're learning

BOSTON — For some people, being recognized as the top gun in your line of work only to find yourself looking for a new line of work a month later might trigger a come-to-Jesus moment — a reexamination of your core principles, how they relate to your profession and how it relates to them. George Karl isn't "some people."
There he was, at the opening panel of the 2014 MIT Sloan Sports Analytics Conference, discussing the in-game applications of research-driven revelations and sounding as adamant as ever. Yes, the longtime head coach whose penchant for pace-pushing produced 1,131 regular-season wins, sixth-most in NBA history, still thinks you should play fast — the faster and looser the better, positional definitions be damned. (For what it's worth, Karl also rolled up an 80-105 career postseason record, with 14 first-round exits in 22 playoff appearances.)
"I just think the game's going to no-position basketball players, quick-decision basketball players and good-decision-making basketball players," Karl said. "We want quick decisions and effective decisions, because when you slow it down, it's not an efficient game."
At this year's Sloan conference, "decision-making" was a hot phrase, joined frequently by "automation" and "machine learning" — the former emblematic of the drive to accelerate (and remove human error from) decision-making, the latter an advancing means for doing it. Coaches aren't the only ones who want quick, effective decisions.
This, of course, makes sense. The annual Sloan conference is where people who care about sports discuss bleeding-edge analysis and new statistical information that could help them learn new things. For attendees who also work for sports franchises, the mission expands to picking up anything — a new metric, a new theory, a new contact, whatever — that could provide even the slightest nudge toward making the best choice, the right call, the decision that couldyou’re your team over the top.
That undercurrent, though, became an overarching theme at Sloan 2014. Three of four finalists in the conference's research paper competition dealt with quantifying and/or exploiting specific in-game decisions:
• A Harvard University group helmed by Grantland's Kirk Goldsberry focused on how the choices NBA players make — whether to shoot, whether to drive, whether to pass, to which teammate — affect the "Expected Possession Value," or "EPV," of an offensive trip at different stages of play.
This could provide a framework for determining which players' decisions are most beneficial (big ups, Jose Calderon and LeBron James) or harmful (sorry, Josh Smith and Brandon Jennings) for their clubs. It could also open the door to ascribing numeric values to things we know matter but have never been able to measure, like entry passes and dribble penetration.
• Etan Green and David P. Daniels of Stanford University showed that baseball umpires have biases behind the plate, displaying an aversion (whether conscious or unconscious) to calling consecutive strikes, while being much more likely to call strikes on three-ball counts and balls on two-strike counts than in other situations.
Savvy batters could benefit from this information by swinging less often after a called strike, while pitchers could profit by, for example, taking advantage of an expanded strike zone in three-ball counts. They mostly don't, the authors say.
• Gartheeban Ganeshapillai and John Guttag of the Massachusetts Institute of Technology sought a better way of figuring out when an MLB manager should replace his starting pitcher with a reliever.
Using a host of factors — score, inning, pitch count, prior-inning performance, how the pitcher has fared against upcoming batters in the past, etc. — they built a model to predict whether a starter was likely to give up a run if allowed to pitch the following inning; if so, it'd yank him. They applied it to data on every pitch thrown from 2006 through 2010 and compared it to managers' actual decisions. In "close game situations" where managers rolled with pitchers the model would've lifted, the pitchers gave up runs in the next inning 60 percent of the time.
"By using machine learning, you can actually build models that could use prove useful in making in-game decisions," Ganeshapillai said.
Ganeshapillai and Guttag weren't the only Sloan presenters whose projects relied on machine learning, a scientific method that's been called "the part of artificial intelligence that actually works." Machine learning became mainstream headline news after a "neural network" proved able to perform an identification task ("learning" how to recognize cats by scanning a database of 10 million images) without human supervision, heralding what The New York Times called an age of computers "able to learn from their own mistakes, a development that is about to turn the digital world on its head."
The method was also employed in two other NBA-related papers, one dedicated to developing a program that can automatically recognize on-ball screens and another aimed at creating a quantifiable definition of "effective shot quality," or "ESQ," by figuring out the most likely predictors that a shot taken by an "average NBA player" will go in.
These efforts, like the EPV project, took the optical tracking data from STATS LLC's SportVU camera system — which was only installed in 15 arenas as of last year, but now hangs in all 29 NBA gyms, creating a league-wide data set for data analysts to dig into — and ran them through some heavy-duty computational machinery to spit out their findings. They met with varying degrees of success; the machines might be learning, but there's still a lot they don't know.
The on-ball screen project had a "positive predictive value" (meaning successful recognition of a screen) of 80 percent. On one hand, 80's pretty good; on the other, one-in-five's a lot to miss, especially when the model's already missing dribble handoffs, which often function similarly to fast-moving pick-and-rolls or brush screens but weren't included in the classification. And failing to capture double screens and staggered screens as separate elements. And unable to account for different personnel groups, which is kind of a big deal, since how a pick-and-roll gets run depends quite a bit on who's running it, who's screening, and who's spotting up around it.
The other machine-learning efforts faced similar shortcomings. The ESQ project — produced by researchers from Second Spectrum, whose optical tracking work on rebounding won top research-paper honors in 2012 and this year — concluded "that it will be difficult to get a model with predictability" higher than 65 percent, putting us far from a reliable touchstone for evaluating healthy shot-taking and shot-making. The EPV effort generalizes players' decisions rather than recognizing that they "in fact execute a carefully designed sequence"; as Goldsberry wrote last month, EPV remains "in its infancy and is by no means going to 'revolutionize' basketball analysis."
The "when to pull your starter" paper misses factors like whether the pitcher is slated to bat the next inning and the options the skipper's got available in the bullpen. It also "doesn’t address [the] scenario" in which a starter leaves mid-inning, which happens a lot. And as the authors note, the work's inherently one-sided; it'll never have information for what would've happened in situations where the model would keep the pitcher in, but the manager takes his guy out. It's easier to seem right when you limit the ways you can be wrong.
These shortfalls don't mean the drive to automate and expedite has concluded, though,; far from it. The more "spatiotemporal" data — the sort of x,y-coordinate information that shows players' location at specific times, what SportVU provides — that analysts get, the more they're going to put it through the computational paces. And not just for the NBA or MLB, either.
A team from Pittsburgh-based Disney Research presented work on a system to automatically recognize how soccer clubs' formations differ between home matches and road games. Advanced Digital Sciences Center research fellow Jagannadan Varadarajan introduced AutoScout, a prototype for analyzing American football aimed at allowing coaches to automatically generate opposing teams' playbooks simply by running opponents' game film through a program that "learns" to identify players, formations and play types by analyzing the routes players take off the snap of the ball. It's still in the early stages, but preliminary testing on clips of live game action resulted in accurately predicted play types about 80 percent of the time.
"We want to run this algorithm on tons and tons of film," Varadarajan said.
The same goes for all of the machine-learning-pushing folks who presented at Sloan. When their models come back with a 65 percent predictability rate, they go back to the drawing board, try again, and come back looking for 70 percent, for 80 percent, for as close to perfection as possible, delivered as quickly as possible. They won't all get there, but they won't stop seeking it, because in the big business world of Big Data sports, information is power and time is money.
"Instead of spending 40 hours retrieving this or cutting tape, you can do it in a second," said Disney Research's Patrick Lucey while presenting the soccer-formation recognition paper.
Reducing a work week into seconds of computation would seem to have enormous value for always-overloaded coaches and scouts … provided, of course, those coaches and scouts trust the info the machines produce.
"You can't take that human element out of the equation," former general manager Bryan Colangelo said during Friday's Basketball Analytics panel.
During that same panel, which also saw Colangelo admit to tanking, former head coach Stan Van Gundy articulated his wariness about accepting data without knowing not only where it came from, but also exactly who produced it.
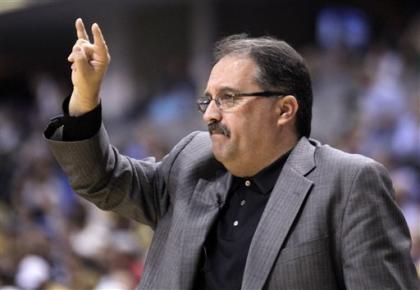
"I read some of the stuff that people write on ESPN.com, and stats on pick-and-roll defense and stuff that came off Synergy [Sports Technology] or somewhere else — I don't know who the hell is recording that information," he said. "Look, a lot of pick-and-rolls — some pick-and-rolls are designed to score, and then there's pick-and-rolls you’re running to get into something else. If you're recording it and treating those two things the same, then you don't know what you’re doing.
"To me, I think that a lot of the analytic stuff can be very useful, but if you're using that in place of sitting down and watching film yourself and seeing what's going on, you’re making a big mistake," he added. "And I don't want to offend anybody, but I think one of the problems with analytics […] is, there are a lot of people in a lot of organizations who don't know the game, who all they know is analytics and, as a result, that's what they rely on. They will use that to supersede what guys like us see with our eyes, and I think that's a major mistake.
"There's no substitute for watching film over and over and over again, and the only numbers I trust are the ones that my people keep."
Given that attitude — which, it's worth noting, is a take shared by many analytically minded writers who have questioned Synergy's game-charting — it seems unlikely that Van Gundy, or coaches like him, would look kindly on a tape breakdown of an upcoming opponent's offense automatically generated by a computer program, even if that program did "watch" every play over and over and over again, and even if the experts who designed it can ratchet the predictability up to a guaranteed 100 percent rate of accuracy. (We now recall Tommy Callahan's NSFW commentary on guarantees.)
I don't know that Van Gundy's approach is shared by the rest of the coaching fraternity. I feel pretty confident, though, in saying that he's not the only one who might look askance at advances intended to not only automate film study and preparation, but also perhaps introduce new decision-making processes into the way coaches operate. (To say nothing of scouts, whom you could see regarding a program that pulls a week's worth of tape study in seconds as a threat.)
Coaches might not raise up like Ron Washington defending the sacrifice bunt when presented with the prospect of ceding some control, but it's not hard to envision them resisting the idea of substituting their own judgment for that of a program. The key for those who design and operate the programs, then? Figuring out how to use the new tools to address the questions the bosses actually want answered.
"If we focus on what they can use to make better decisions, then the barriers to communication will just disappear," said Benjamin Alamar, a professor and sports analytics consultant whose work with pro teams includes five years with the Oklahoma City Thunder. "We need to think about what our decision-makers — our coaches, our general managers — think about."
Mostly, that's how to win games and how to keep their jobs. If the analysts behind stuff like EPV, ESQ and AutoScout can show coaches and GMs that what they're offering helps on scoreboards and in standings, they'll get a voice in the process and a seat at the table. That day isn't here yet, but it might be soon. There's a lot they don't know, but the machines are learning.
More from the 2014 MIT Sloan Sports Analytics Conference:
• Former Toronto Raptors GM Bryan Colangelo admits to tanking ... or, at least, trying to
• Adam Silver weighs in on NBA draft age limit, tanking, potential lottery and postseason changes, and more
Check out what's buzzing on the Yahoo Sports Minute:
- - - - - - -

is an editor for Ball Don't Lie on Yahoo Sports. Have a tip? Email him at devine@yahoo-inc.com or follow him on Twitter!
Stay connected with Ball Don't Lie on Twitter @YahooBDL, "Like" BDL on Facebook and follow BDL's Tumblr for year-round NBA talk, jokes and more.